If you’re a data scientist, you probably recognize the names DJ Patil and Jeff Hammerbacher. Not only are both often credited with popularizing the term “data science,” but they also exemplify the modern data scientist—that is, one who applies his or her data-savvy expertise in any setting that demands it, including healthcare, e-commerce, social media, and journalism—just to name a few.
Patil, the chief data scientist at the United States Office of Science and Technology Policy, boasts an extensive resume that includes stints at LinkedIn, Greylock Partners, Skype, PayPal, and eBay.
Hammerbacher is cofounder of Cloudera, a software company that provides Apache Hadoop-based software, support and services, and training to business customers. His resume is also peppered with experience working for big-name companies such as Facebook, O’Reilly Media, CIOX Health, and others.
As experienced data scientists working in a variety of industries, their stories are certainly not unique. However, their careers would have taken very different trajectories had they been employed long before the discipline became what Harvard Business Review dubbed as the “sexiest job of the 21st century.”
Pushing Rewind on Data Science
Although data science isn’t a new profession, it has evolved considerably over the last 50 years. A trip into the history of data science reveals a long and winding path that began as early as 1962 when mathematician John W. Tukey predicted the effect of modern-day electronic computing on data analysis as an empirical science.
Yet, the data science of today is a far cry from the one that Tukey imagined. Tukey’s predictions occurred well before the explosion of big data and the ability to perform complex and large-scale analyses. After all, it wasn’t until 1964 that the first desktop computer—Programma 101—was unveiled to the public at the New York World’s Fair. Any analyses that took place were far more rudimentary than the ones that are possible today.
By 1981, IBM had released its first personal computer. Apple wasn’t far behind, releasing the first personal computer with a graphical user interface in 1983. Throughout that decade, computing seemed to evolve at a much faster pace, giving companies the ability to collect data more easily. However, it would be nearly two decades before they would start to convert that data into information and knowledge.
A New Era of Data Science
Throughout the 2000s, various academic journals began to recognize data science as an emerging discipline. In 2005, the National Science Board advocated for a data science career path to ensure that there would be experts who could successfully manage a digital data collection.
By this time, companies had also begun to view data as a commodity upon which they could capitalize. Thomas H. Davenport, Don Cohen, and Al Jacobson wrote in a 2005 Babson College Working Knowledge Research Center report, “Instead of competing on traditional factors, companies are beginning to employ statistical and quantitative analysis and predictive modeling as primary elements of competition.”
Still, in 2009, Google Chief Economist Hal Varian told the McKinsey Quarterly that he was concerned with the deficit of individuals qualified to analyze the “free and ubiquitous data” being generated. He said, “The complimentary scarce factor is the ability to understand that data and extract value from it … I do think those skills—of being able to access, understand, and communicate the insights you get from the data analysis—are going to be extremely important.”
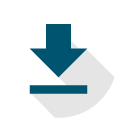
Get Program Guide
Learn more about our 100% online degree and certificate programs.
Data Science in Demand
Today’s data scientists know Varian was right. The good news is that in 2010, all of that started to change as data science began to take center stage against the backdrop of significant advancements in computing technology.
For example, Apple introduced the iPad in January 2010. In June of that same year, Apple released its fourth-generation iPhone. Consumers began to embrace technology—particularly mobile technology—at lightning speed. In July, Amazon published a press release stating that for the first time ever, it had sold more Kindle books than hardcover books.
With faster processing speeds than ever before, technology took a giant leap into the new decade, blazing a trail for individuals ready and willing to conquer the mountain of big data that had only begun to grow.
Data Science Continues to Grow
Over the last few years, data science has continued to evolve and permeate nearly every industry that generates or relies on data. In an article from Spiceworks, Kareem Bakr deems data scientists the “hottest talent pool in 2023.”
“Data science hiring is poised to dominate over the next year as candidates with the skills will be entering a favorable, skills-driven market and is one of the most industry-agnostic pools of talent as their expertise is needed across industries like pharma, finance, and supply chain,” Bakr writes.
Today, data scientists are invaluable to any company in which they work, and employers are willing to pay top dollar to hire them. Also, data science degree programs have emerged to train the next generation of data scientists.
Increasingly, data scientists are also drawn from a variety of different academic and professional backgrounds, including health information management, computer science, and psychology.
Our guess is that data science and its applications will only continue to grow. That’s because big data will become even bigger. For example, 97 percent of Americans now own a cell phone of some kind, according to the Pew Research Center. Nearly eight in ten U.S. adults own desktop or laptop computers, while roughly half now own tablet computers and around one in five own e-reader devices. In addition, one in five Americans use smart watches or fitness trackers.
Learning from the Past
History teaches us many lessons, and that’s certainly true in data science. Here’s what we can learn from the history of data science:
- Don’t take the data for granted. There was a time when data wasn’t nearly as accessible as it is today, nor were people as willing to share it freely. This doesn’t negate the fact that privacy and other ethical concerns remain, and data scientists must know how to operate within an ethical framework as the tsunami of data grows. And even though data is more accessible, much of it remains unstructured, paving the way for new methods of analyses.
- Think big. Big data requires big analyses, and as technology evolves, data scientists must evolve their high-performance computing skills as well. This includes the ability to perform complex data mining and prescriptive analytics.
- Know the context. Unlike in the past when data scientists worked primarily in the information technology sector, today’s data scientists work in a variety of industries, helping organizations make data-driven decisions that change the way in which they compete in the larger marketplace. To be successful, data scientists must be well-versed in data communication and strategic decision-making.
Data science will undoubtedly continue to evolve as industry needs change. However, one point remains clear: Data scientists will always be in demand. As long as data exists, there must be highly skilled individuals who can analyze it. The questions for the future are, just how much data will be available, where will it come from, and what new analysis techniques will have emerged by then to give us even greater insights?
More Data Science Stories
How to Make Your Data Science Resume Stand Out
Data Science Student Pursues Data Analysis in Anticipation of ‘Industry 4.0’ Smart Factories